Phase II: Semisupervised Learning Enabled Hospital Readmission Prediction and Magnetic Resonance Angiography Heat Mapping
Phase 2 involves combining the Framingham Heart Study Dataset with a Hospital Readmission Prediction (Kaiser Permanente) dataset using Semi-Supervised Learning (SSL).
The Hospital Readmission Prediction dataset shares some features with FHS. The semi-supervised learning (SSL) approach combines the data from those select shared features to generate values for non-shared features (These generated values are "Pseudo-Labeled").
From there, the model is trained on the combined Labeled and Pseudo-Labeled aggregated dataset. Finally, the probability of a patient being readmitted to a hospital and the risk of contracting CHD in 10 years is output, with the input being a limited amount of clinical/social data.
​
​
Phase 3 involves using Saliency Mapping, a Computer Vision technique that shows heat spots or regions of interest when a CT or MRA comes in. Specifically, it colors the individual pixels based on their contribution to the classification.
These heat spots are segmentations of the areas that physicians should pay attention to. I intend to use Saliency mapping on my dataset of classified Magnetic Resonance Angiography images from the Sunnybrook Cardiac Dataset for the time being (Although I can use the model on the MESA Study Dataset if/when I get access to it).
From there, I plan to compare these results with manual annotations from 2 licensed cardiologists, Dr.Tassiopoulos and Dr.Yeddanapudi to evaluate their legitimacy.
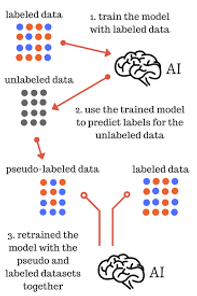
Semisupervised Learning
Demonstration
